What Do You Speak For?
- Mike Cisneros
- May 11, 2017
- 5 min read
(Charlie Hutcheson has written a fine technical review of my viz for this week as part of his Takeapart Tuesday series. I encourage you to read it, and all of his posts. For myself, I wanted to write about the “why” of this week’s viz, rather than the “what.”)
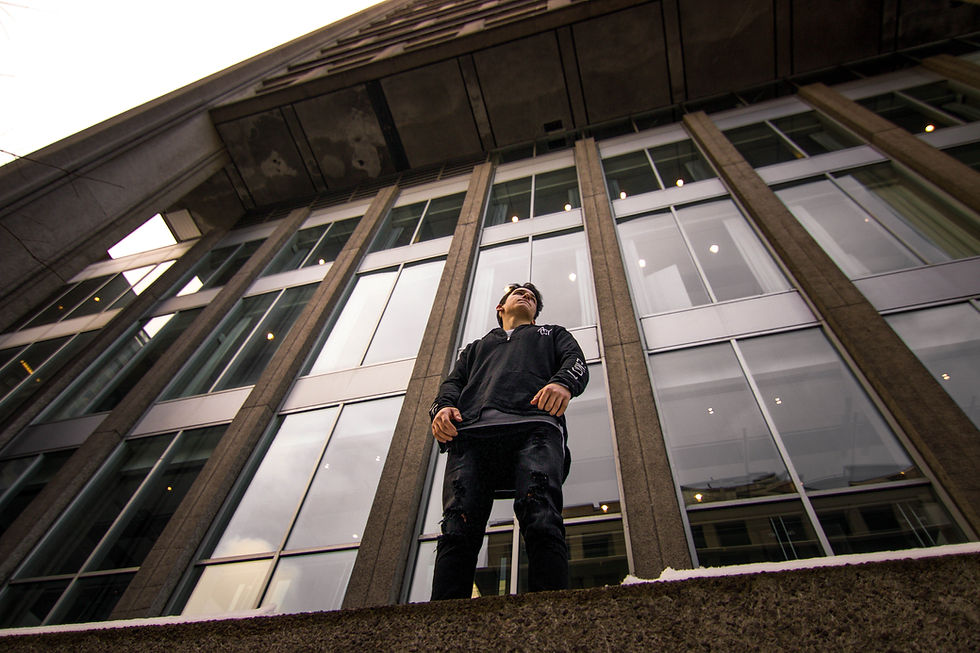
I don’t presume to know what was inside the heads of the Andys when they started #MakeoverMonday in 2016, but I believe their intentions were largely
to engage the critical thinking of the Tableau Public community,
by highlighting data visualizations in-the-wild that were less than optimally designed for their specific tasks, and
challenging people to “makeover” said visualizations in a more clear, communicative, or persuasive fashion.
As the project evolved, guidelines and suggestions both faded and arose.
The original “one-hour timeboxing” suggestion seems mostly disregarded nowadays (I certainly disregard it).
An increase in the frequency with which participants would incorporate secondary data sets ultimately led to an explicit directive to only use the provided data…a directive that has since been (more or less) lifted.
The very premise of the original effort—remake an existing viz, ideally retelling its story in a more effective way—is no longer assured, as there have been several weeks where participants were provided only a data set, and no baseline visualization whatsoever.
This last change actually makes #MakeoverMonday a more interesting, complex, tricky, and possibly contentious project. Originally, participants had the cushion of a specifically prescribed data set, a baseline visualization, and a mandate only to redo what they saw. Now, the possibilities are much wider – especially since secondary data sets are once again “allowed” to be included – and as such, so are our responsibilities to the data.
What Do You Mean By That, Killjoy?
It means that we are getting more, and better, data sources to work with, by and large, than we were in the past. But that also means that we have to do a lot more legwork before embarking upon any kind of visualization, in order to ensure that we fully understand what that data actually is.
A cavalier attitude towards certain data sets—and beyond that, the means by which those data sets were collected—has already led to a number of discussions this year alone (see “Week 1: Australia's Gender Pay Gap,” “Week 3: Trump’s Tweets,” and “Week 13: The Secret of Success” for weeks where data integrity or collection methods were debated assiduously).
Yes, it’s a pain in the ass to have to put a lot of effort into thoroughly vetting and validating giant data sets, especially when it’s a “just-for-fun” project. But if the work you’re doing is going to hit the public eye IN ANY WAY, including but not limited to:
being posted on your Tableau Public page;
being shared on Twitter as a screenshot or as a linked viz;
being blogged about, by yourself or someone else;
being featured in a weekly wrapup; and/or
being a Viz of the Day
...it’s incumbent upon you to make sure that your visualization is representing the data accurately.
“My Job is to Speak for the Data”
Here comes yet another appearance of my impressive wife in my blog. As a biostatistician, she was often, while at her previous employer, asked to serve on Data Monitoring Committees (DMCs) for clinical trials. There are complexities involving who on the committee can see what information at what time, but essentially, these are the people who evaluate the progress of pharmaceutical trials throughout their duration, and ultimately will be able to say how successful a trial has been—and, more importantly, if the trial is still safe enough to continue.
People in the position that my wife has are often in a difficult role; although they are hired by the pharmaceutical company running the trial, they can end up costing that company hundreds of millions of dollars, simply by reporting that a trial is no longer safe to continue—that is, by performing their jobs with integrity and honesty.
She would describe her job as “speaking for the data,” meaning that regardless of who paid for her work, the job entailed looking at the objectively reported data, making statistically accurate assessments and recommendations, and preventing the data from being misrepresented. (As always, “the data” actually means “people who are taking an experimental drug and reporting the effects,” so it is critical to have a strong, impartial advocate for “the data.”)
We should all strive to “speak for the data” as honestly and as accurately as a biostatistician tasked with ensuring that people aren’t dying unnecessarily from an experimental drug.
Cars in the Netherlands
Wanting to speak for the data accurately is why I chose my specific approach to the Dutch cars dataset.
I knew the data didn’t actually say when cars were bought, or sold, or how much they were bought or sold for.
I also knew that the only registrations captured in the data, for any given car, were the first one, and the most recent one. (If the car were originally from outside of the Netherlands, it would also capture the “first registered in Netherlands” date.)
Some of the records have a “license expiration date” value; most of them are null. According to the internet research I was able to do, Dutch licenses don’t seem to expire, so this one was a bit of a head-scratcher.
Nevertheless, I felt like I couldn’t in good conscience use this data to make many big-picture assessments, other than possibly to see how many most recent registrations were from the same year as the very-first-NL registration.
Turns out, roughly 30%! Is that a lot? I don’t know! Feels like it! But that’s just the thing: I DON’T KNOW, so I shouldn’t say that it is or it isn’t. In this case, I should just present the number.
I also found that there’s a weird bump in registrations in 1995 for cars originally registered from 1985 and earlier. Why? I DON’T KNOW. And I tried to find out, but it’s really hard to search for specific 20-year-old regulatory minutiae in a foreign language. (Now, I know a beginner’s level of Dutch—like, restaurant-and-hotel-and-street-sign level Dutch—but not nearly enough to figure out the intricacies of EU regulations.)
I also think it’s awfully strange that no matter what year of car you have, as long as it’s 2007 or older, your most likely year of registration is 2016. I know that the Netherlands are changing their registration cards from paper to plastic credit-card-style cards, and that it started in 2014 and will be required by 2019; and I suspect that people are re-registering their cars in order to be compliant with this policy, but do I know that for sure? NO I DON’T! I can only speculate.
So as much as I want to be clever and “A-HA!” annotations all over the viz, and write clever titles and bump up the virality of this incredibly groundbreaking </sarcasm> data analysis…mostly I can’t. I can speak for the data: show what the numbers say. But I can’t go one single step beyond that into Presumption Land. That’s when I would be crossing the line from analyst into pundit.
Fallibility and Inevitability
Look, I’m sure there are elements of this viz that can be easily explained. There may well be pieces of it that I’m misunderstanding. But I feel that I’ve done the best I can to avoid the obvious traps, and tried very hard not to make pronouncements that the data do not support. Where I had suspicions, I labeled them as suspicions. What I didn’t do is try to force the data to fit a narrative. I let the data tell me what the narrative is—could POSSIBLY be—and if that’s, well, kind of boring, so be it.
Sometimes—in fact, lots of the time—a new customer will present you a data set with the directions, “Tell me what interesting things you can find here,” and the honest answer, unfortunately for you and your potentially ongoing financial relationship with your new client, is, “There’s nothing interesting here.” In that moment, it’s hard to make that assessment. But swallow hard and do it, because that’s your job.
Speak for the data.
Comments